Description
"Numerical modelling of subsurface flow in the context of gas storage often faces the challenge of long time periods and large spatial domains. Therefore, computational models that are robust, fast and give an accurate prediction of the system are desired, e.g., to help investigate potential gas storage sites, determine optimal operational parameters, and ensure safety of operation. The most efficient model at a specific time during the simulation or at a specific location in the domain can be used by coupling models of different complexity (multiphysics or hybrid model). Resulting multiphysics models are expected to be robust and computationally efficient on domains with varying complexity because they can adaptively match model complexity to domain/process complexity for different times during the simulation and different parts of the domain.
The goal of this project is a fully-implicit hybrid model with local dynamic model adaptivity employing machine-learned scale-bridging relations for the description of gas-storage scenarios. The hybrid multi-scale model couples a full-dimensional fine-scale model with a vertical-equilibrium coarse-scale model. In order to overcome the severe time-step limitations posed by the sequential, i.e., IMPES-type, solution approach developed in the precursor project, we will pursue a fully-implicit approach. This requires the determination of the transition region before each time step where a model switch is allowed during that step. Solution strategies developed in the project network for the systems of nonlinear and linear equations will be assessed and developed further. The benefit of the fully-implicit model compared to the sequential one will be analyzed. As they constitute a significant part of the computational effort, the necessary scale-bridging calculations will be optimized by machine learning. The model will be vali- dated against experimental data by employing a Bayesian framework, taking into account uncertainties in a rigorous way and offering the possibility to quantify the effect of competing modeling choices."
Project leader
apl. Prof. Dr. rer. nat. Bernd Flemisch
Researcher
Ivan Buntic (M.Sc.)
Partners
Prof. Dr. Ingo Steinwart
Duration
09/2023 - 12/2025
Contact
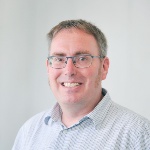
Bernd Flemisch
apl. Prof. Dr. rer. nat.Professor for Simulation Technology
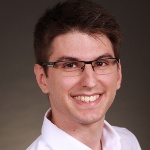
Ivan Buntic
M.Sc.Academic Staff